Last updated: June 15, 2023
Article
Eavesdropping (On Birds) Has a Smart New Tool
BirdNET uses artificial intelligence to analyze audio recordings and detect bird species by sound. We’re exploring its potential to help parks answer complex, pressing questions.

Image credit: NPS / Cathleen Balantic
One sunny Friday afternoon in 2015 found me hunched over my laptop in a quiet office in Southern California. My colleagues and I had just spent six months turning smartphones into audio recorders for monitoring wildlife sounds remotely. At the time, I wasn’t sure our idea would work. An entire spring and summer had been consumed by testing microphones and cables, learning how to solder electronics, and baking smartphones in an oven. This last was to figure out their heat tolerance. We also cobbled together enough YouTube-based insights to learn how to make the phones turn on by themselves when connected to power.
It had been an ordeal just to get everything working on my porch in Vermont, so I anxiously anticipated the challenges awaiting us in the California desert. On the way over, my connecting flight was canceled. I slept on the airport floor while hugging my precious bag of heavily modified budget smartphones. I churned through fitful dreams of all the ways our project could go awry.
Our inventions—each tucked away in a weatherproof case with a solar panel for power—were now on their own in the wild, automatically recording sounds.
By that sunny Friday, though, our field work had been completed with few hiccups. We’d installed our devices at various sites throughout the desert. Our inventions—each tucked away in a weatherproof case with a solar panel for power—were now on their own in the wild, automatically recording sounds. Once the devices collected the audio files, they sent them over a cellular network to my laptop, about a hundred miles from the monitoring sites.
I opened one of the audio files and heard one of our target species: a black-tailed gnatcatcher. It was the first bird we captured on our do-it-yourself remote audio recorders. Hearing that bird was my equivalent of the scene at the end of a space movie where the control room erupts in cheers after saving the crew. Our acoustic monitoring project was actually going to work.

Image credit: NPS / Jane Gamble
Big Data Dilemma Solved—except for One Elusive Bird
Many bird species are easy for people to identify by ear based on the sounds they make. So if you want to know which birds are around you, installing audio recorders at sites of interest can be a good way to do it. Audio recordings not only give a snapshot of which birds are present, but also can outperform human observers at this task. The resulting acoustic record allows us to ask questions that go beyond whether species are present or absent: How are bird populations responding to climate change? How do animals alter their calling behavior in the presence of noise?
As hundreds of hours of audio rolled in over the subsequent year of our desert monitoring project, we encountered an obvious challenge: there was no way for us to listen to all of it. How, then, could we figure out which birds were actually on the recordings? Other researchers have described similar difficulties and have tackled this problem using software tools like the R package monitoR, Raven Pro, or OpenSoundscape. Though the specifics may vary, the general answer is the same: we use an automated detection system to make the computer do the listening for us.
We learned a lot in that project, but not much about black-tailed gnatcatchers.
But black-tailed gnatcatchers have harsh, raspy calls that were too difficult to detect reliably with the automated system I was using at the time. My options were to go through the files manually in search of gnatcatcher sounds or build a much more specialized type of detection system, which I lacked the expertise to do. After many failed attempts to create an automated detector that could consistently identify this bird’s sound, I gave up. I decided to work on other birds whose sounds were easier to detect with the tools I already had.
In the following year, after much tuning and tinkering, I managed to build successful automated detectors for several other bird species. Our detectors raced through hundreds of hours of audio at over a dozen monitoring sites. They located the sounds of verdin, Gambel’s quail, and Eurasian collared-dove with ease. We now understood where these species might be found and when we can expect to hear them. We learned a lot in that project, but not much about black-tailed gnatcatchers.

Image credit: NPS / Therese Donovan
BirdNET Catches More Species, and Quickly
Back in 2015, I was a PhD student working on one project and a handful of species in a single habitat . Now I’m a biologist in the National Park Service’s Natural Sounds and Night Skies Division. Parks seek us out to help address a variety of issues around noise and light pollution. We also help answer questions about wildlife sounds.
I can build an automated detector for a target species in a park, and I can often craft it well enough to detect that species reliably. This is a fine solution for a single monitoring project, but it’s not a good method for projects in multiple parks. Each park has different target animals, soundscapes, and management needs. The needs and questions multiply very quickly, and so does the time involved in answering them. Instead of painstakingly building automated bird sound detectors for individual species the way I used to, I’ve been investigating a new tool called BirdNET.
BirdNET is a free, open-source, automated program that uses artificial intelligence to detect over 3,000 bird species. You can download it onto your computer and run audio recordings through it to get a time-stamped list of the bird species in the recordings. The program also tells you how certain it is that it identified the right species.
As someone who has spent hundreds of hours building automated detectors for different projects, this is groundbreaking.
As someone who has spent hundreds of hours building automated detectors for different projects, this is groundbreaking. BirdNET might help scientists skip a very time-consuming part of the process—creating detectors for target species—and instead focus directly on answering complex but essential questions.
But like any model, BirdNET has limitations. A golden-crowned kinglet detected with 10 percent confidence could be a false positive, as the low confidence value suggests. To avoid false positives—claims that a particular species was heard when in fact it was not—should we ignore BirdNET’s low confidence detections and only focus on high confidence detections? Perhaps. But if we only keep high confidence detections, we might miss out on a varied thrush song detected with only 38 percent confidence. If the species was truly vocalizing, throwing away this low confidence score would create a false negative.
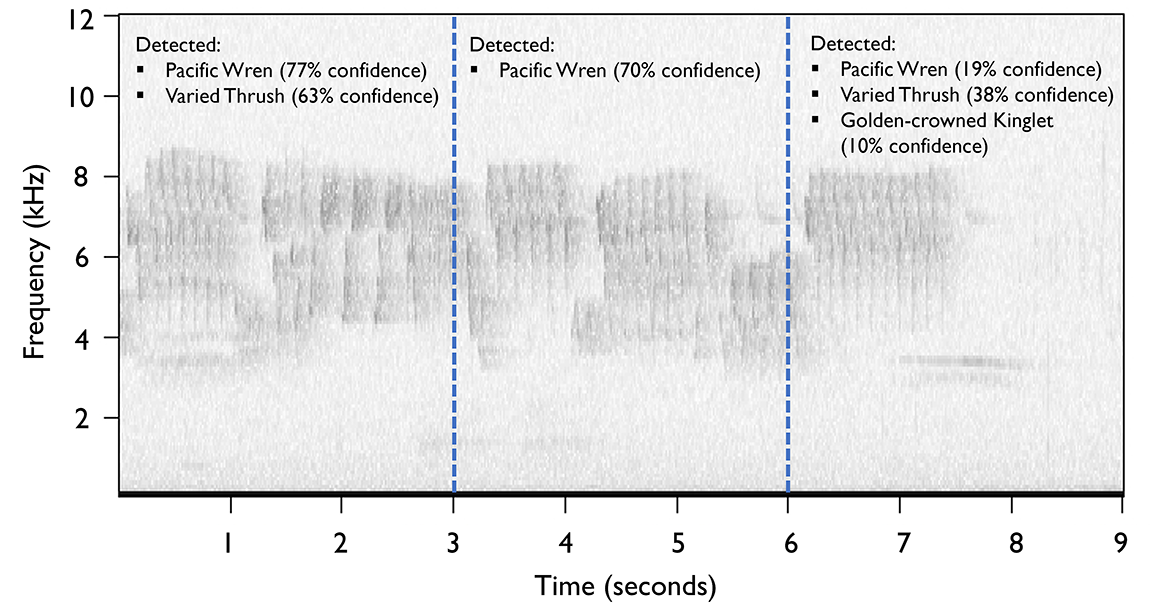
Image credit: NPS / Cathleen Balantic
-
BirdNET audio from Bartlett Cove, Glacier Bay National Park and Preserve, spring 2022
A Pacific wren sings from 0 to 7.5 seconds. A varied thrush sings at approximately 0 to 1 seconds and 7 to 8 seconds. Another species, possibly an American crow, vocalizes at around 3 to 4 seconds. From "Eavesdropping (on Birds) Has a Smart New Tool," Park Science magazine, Winter 2022 issue (December 30, 2022).
- Credit / Author:
- NPS / Cathleen Balantic
- Date created:
- 12/20/2022
BirdNET isn’t perfect, and it doesn’t claim to be. As with any model, there is uncertainty involved, and its detection capabilities are likely to vary by species and location. But it’s the most powerful off-the-shelf tool I know of for detecting birds in huge audio datasets. So how do we aggregate the wealth of uncertain information BirdNET offers into something that a scientist or park manager can use?
Could This AI Help Answer Tough Questions?
We’ve been using BirdNET to assess how the avian soundscape is changing over time in a long-running acoustic monitoring project at Glacier Bay National Park and Preserve. Our initial findings suggest that the makeup of bird sounds in the park is different in recent years compared with a decade ago. Are we hearing the consequences of climate change, or are there other variables at play? We’re not sure yet, but combining climate data with BirdNET detections may help us learn more. We’re conducting a similar acoustic monitoring project at Lewis and Clark National Historical Park—about a thousand miles southeast of Glacier Bay as the pacific wren flies. Together, these two sites may give us an idea of how soundscapes are changing along the Pacific flyway.

Image credit: U.S. Fish and Wildlife Service / Kristine Sowl
We’ve also been investigating what bird sounds can tell us about park management. Rocky Mountain National Park has installed simulated beaver structures at several streams to restore degraded meadow ecosystems. We’re monitoring those sites using audio recorders. Our preliminary results suggest that bird biodiversity is higher at sites with the simulated beaver structures than at sites without them. There’s still a lot to untangle in this story, but BirdNET has enabled us to learn about these sites quickly. We’ll be able to ask more precise questions as the project continues.
One of the most promising uses of BirdNET is for monitoring bird presence or absence. In a project at Tule Springs Fossil Beds National Monument, BirdNET is giving us an inkling of which species might be at different park locations throughout the year. This is particularly important for species whose numbers have declined or who are otherwise at risk. The uncertainty involved in automated detection means we need to interpret early results with caution. What’s clear, though, is that the ability to conduct rapid species assessments with BirdNET may help us tackle important scientific and management questions.
Dreaming Big
There’s a lot more we can do to maximize the utility of BirdNET for national parks, and it’s fun to dream big. One challenge is making the software easier for park scientists to use. We’ve been working on that but have a long way to go. I’m also dreaming about a platform that might allow volunteers, park biologists, visitors, or local birders to help verify BirdNET detections in park sound recordings.
Similar efforts are already underway. Citizen science seems to be a priority for the BirdNET team. Initiatives like BirdNET’s live submission map, BirdNET-Pi, and BirdWeather illustrate how powerful and widely adopted this open-source technology could become. Sophisticated tools like BirdNET can help us answer pressing questions about climate change, conservation, and ultimately, whether park management strategies are working.

About the author
Cathleen Balantic is a biologist with the National Park Service's Natural Sounds and Night Skies Division. She specializes in bioacoustics. She works on projects that use biological sounds to learn about wildlife in our national parks. Image credit: NPS / Cathleen Balantic
Tags
- glacier bay national park & preserve
- lewis and clark national historical park
- rocky mountain national park
- tule springs fossil beds national monument
- birdnet
- birds
- artificial intelligence
- tracking
- natural sounds
- cathleen balantic
- animals
- perspectives
- ps v36 n2
- park science magazine
- park science journal
- stories by women scientists
- stories by women authors