Last updated: September 11, 2024
Article
Wildlife Monitoring at Saguaro National Park, Tucson Mountain District: 2023

NPS
Overview
The National Park Service Sonoran Desert Inventory and Monitoring Network uses passively triggered remote wildlife cameras to monitor medium-sized and large mammals at the Tucson Mountain District of Saguaro National Park. In January and February of 2023, the Sonoran Desert Network deployed 59 wildlife cameras, which collectively captured 3,967 photos of mammals. We limited our analyses to the six weeks when most cameras were active. During those six weeks, we obtained 3,746 photos of mammals and detected 13 mammal species and 1 mammal genus (Lepus) that could not be identified to species (see results section below for more information). Our cameras also captured birds, reptiles, and amphibians, and while some of these images are shown in the photo gallery below, we only summarize findings from mammal monitoring in 2023 for this report. Long-term, multi-year data will be analyzed to investigate changes in mammal use of habitat on the park and will be reported elsewhere.
Monitoring changes in wildlife populations over time, including identifying the effects of environmental and human-caused factors on wildlife populations, gives us valuable information for managing parks to protect wildlife. Remote camera data can tell us what park habitats are being used by wildlife and how that use varies over time. Analyzing this information in conjunction with environmental variables allows us to examine the factors that may be driving wildlife distribution patterns. We used single-season occupancy models to analyze the 2023 wildlife monitoring data in the Tucson Mountain District of Saguaro National Park, and we present those results in this report.

NPS
Background
The distribution of many wildlife species may change in response to disturbances, such as habitat loss and climate change. Large, protected areas, such as national parks, can provide critical wildlife habitat—but even they are susceptible to human-caused disturbances (Brashares 2010; Carroll 2010; Chen et al. 2022). Knowing how wildlife species respond to various pressures requires an understanding of animal distribution, multi-species interactions, and abiotic factors at broad spatial scales (Post et al. 2009).
Remote wildlife cameras are powerful research tools that are noninvasive and allow researchers to collect data on multiple species simultaneously over large spatial scales. We use occupancy modeling of remote camera data and environmental covariate data to identify trends in terrestrial mammal populations and better understand the pressures that affect mammal distribution across the landscape.
Commonly, detection data from wildlife cameras are used to estimate occupancy, or the probability that a species occurs in (or “uses”) a particular area. When occupancy probabilities (probabilities of use) are aggregated over a larger area, like a national park, it is possible to estimate the proportion of the larger area that is used by the species. However, photos from wildlife cameras provide imperfect information about a species’ use of that area. If the species is detected (positively identified in one or more photos) at a given camera location, we know the species is present in that area. However, if a species is not detected at a given location, we cannot be sure that the species is truly absent; the species may be present but the camera failed to obtain a picture of that species that year. Occupancy models (MacKenzie et al. 2002) are powerful tools that allow us to estimate probabilities of use and how they might vary spatially using data from wildlife camera photos, all while accounting for imperfect detection.
In addition to estimating occupancy probabilities, we can also use these models to identify landscape features (e.g., elevation, slope) that influence patterns of use and other variables (e.g., day of the year) that could influence the likelihood of detecting a species if it is present (i.e., detection probability).
Methods
The Sonoran Desert Network mammal monitoring methods are based on the peer-reviewed, international protocol for monitoring terrestrial mammals developed by the Tropical Ecology Assessment and Monitoring Network (TEAM 2011).Field Methods
We deployed non-baited wildlife cameras at pre-established locations throughout the Tucson Mountain District of Saguaro National Park. The cameras have been deployed annually during the same time period and roughly in the same locations since 2017 (except for 2019 because of the government shutdown). The cameras were deployed near grid midpoints on a systematic grid covering the Tucson Mountain District. Midpoints were spaced one kilometer apart, but cameras were not deployed at all midpoints. The same midpoints are sampled each year. Crews use their judgement to select the best camera placement and angle to capture photos of mammals (Hubbard et al. in prep). Wildlife cameras use infrared and motion-trigger technology. They automatically take a photo when there is motion or a sudden temperature change in the camera detection zone. Cameras collected data for roughly six weeks before retrieval.
Photo Identification
At the Desert Research Learning Center, biotechnicians analyzed the contents of each wildlife camera memory card. This process began by downloading the photos and using v4.1.0 of Megadetector (Beery et al. 2020), an object detection model designed for camera trap images. We used a confidence level threshold of 0.8 to remove “false triggers,” which are photos that do not contain an animal. The remaining photos were then loaded into the Colorado Parks and Wildlife Photo Warehouse Database and two Sonoran Desert Network observers identified the species in each photo. Observers did not count or try to distinguish between individual animals. Any species identification disagreements were resolved by a referee (Ivan and Newkirk 2016).
Single-Species, Single-Season Occupancy Models
For each of the seven species that had enough detections to analyze occupancy, we used single-season occupancy models to estimate the probability of occurrence and detection probabilities (MacKenzie et al. 2002). First, we defined the season as the continuous period between the first deployment date and the last retrieval date when ≥ 60% of cameras were operational. We then delineated consecutive 7-day sampling occasions within the season starting with the first day of the defined season. For each species and camera location, we generated encounter histories, where a “1” denoted that the species was detected at least once during a sampling occasion and a “0” indicated that the species was not detected. For example, an encounter history of “10011” would indicate that a species was photographed at least once during the first, fourth, and fifth sampling occasions and was not photographed during the second and third sampling occasions.
We used a Bayesian framework to estimate model parameters, as this made it easier to estimate derived parameters (e.g., proportion of area occupied across the entire park) with associated uncertainties. We fit models in R using the spOccupancy package (Doser et al. 2022). For each model, we ran 3 Markov chains, initiated at random values, for 8000 iterations. We discarded the first 4000 iterations (the burn-in) and retained 1 of every 8 iterations thereafter, using the remaining 1500 samples (across all the chains) to summarize the posterior distribution. We verified that the effective sample sizes for model parameters were > 400.
Covariates and Model Selection
For each species we modeled, we first evaluated whether day of the year (linear and quadratic terms), effort (proportion of days during each sampling occasion that the camera was operational), or the experience of the person deploying the camera influenced detection probability. Personnel were categorized as novice (< 1 year experience), experienced (1–2 years of experience), or expert (2+ years of experience). We identified several topographic (aspect, elevation, slope), vegetation-related (vegetation classes and distance to nearest wash), and human-caused (distances to nearest road, park boundary, trail, and point-of-interest) spatial covariates for the Tucson Mountain District of Saguaro National Park that could explain variation in occurrence probabilities. We then assembled a set of candidate models where each model included the best detection covariate(s) and one element from each category of occurrence covariates, excluding any combination of covariates that were highly correlated (pairwise correlation coefficient, |r| > 0.7). We used widely applicable information criterion (WAIC) to identify the best supported candidate model and then removed any covariates with little to no explanatory power (f < 0.9; where f represents the proportion of posterior samples that were < 0 if the posterior mean was < 0, or the proportion of posterior samples > 0 if the posterior mean was > 0). When necessary to estimate spatial variation in occupancy or detection, we included camera location as a random effect. We used the resulting model for all inferences.

NPS
2023 Results
Effort
A total of 59 cameras (Cuddeback G-5017) were deployed in the Tucson Mountain District of Saguaro National Park between 5 January and 22 January 2023 and were retrieved between 23 February and 26 February 2023. We delineated a total of six sampling occasions that were each seven days long. The first sampling occasion began on 12 January 2023 and the last sampling occasion ended on 22 February 2023. Community scientists, park staff, and partner organizations typically assist with camera deployments and retrievals. In 2023, nine volunteers, one international volunteer, and two park staff helped deploy and retrieve cameras in the Tucson Mountain District of the park. We are grateful for their support.
Detections
We observed a total of 13 mammal species plus one mammal that could only be identified to genus (Lepus) in 3,967 photos from the 59 cameras during the 2023 wildlife camera deployment. The mammal genus Lepus includes two species of jackrabbits that are known to be present in the Tucson Mountain District: black-tailed jackrabbit (Lepus californicus) and antelope jackrabbit (Lepus alleni). We did not detect any antelope jackrabbits, but there were 247 photographs that could only be identified to the Lepus genus because the images were not clear enough to distinguish between these two jackrabbit species.
During the six 1-week sampling occasions in 2023, we recorded 3,746 detections. All mammal species listed in Table 1 were observed and detected during previous Sonoran Desert Network wildlife monitoring in the Tucson Mountain District. We detected several species of interest in the “Lost Carnivores” project: American badger, ringtail, bobcat, American hog-nosed skunk, and spotted skunk. The Lost Carnivores project focuses on the Tucson Mountains, including areas outside Saguaro National Park. Learn more about the Lost Carnivores project at their website.
Common Name | Scientific Name | # of Photos | # of Detections | # of Locations |
---|---|---|---|---|
collared peccary | Pecari tajacu | 809 | 75 | 38 |
desert cottontail | Sylvilagus audubonii | 782 | 113 | 26 |
mule deer | Odocoileus hemionus | 757 | 97 | 38 |
black-tailed jackrabbit | Lepus californicus | 676 | 92 | 25 |
coyote | Canis latrans | 353 | 94 | 31 |
unknown jackrabbit | Lepus spp. | 247 | 50 | 15 |
common gray fox | Urocyon cinereoargenteus | 234 | 81 | 34 |
mountain lion | Puma concolor | 47 | 3 | 3 |
bobcat | Lynx rufus | 41 | 21 | 12 |
rock squirrel | Otospermophilus variegatus | 10 | 5 | 3 |
American badger | Taxidea taxus | 6 | 4 | 3 |
ringtail | Bassariscus astutus | 3 | 3 | 2 |
American hog-nosed skunk | Conepatus leuconotus | 1 | 1 | 1 |
western spotted skunk | Spilogale gracilis | 1 | 1 | 1 |
Single-season Occupancy
collared peccary ǀ desert cottontail ǀ mule deer ǀ black-tailed jackrabbit ǀ coyote ǀ gray fox ǀ bobcatCollared peccary (javelina)
Collared peccaries (javelina; Pecari tajacu) were more likely to use hilly or foothills areas and more likely to use areas away from roads within the Tucson Mountain District in 2023 (Figure 1). The southeastern and far northern portions of the district mainly consist of hills and foothills, and roads are sparse, which results in the highest predicted probability of javelina occurrence within the district (> 0.8). However, occurrence probability decreases as the hills and foothills are closer to roads in the west-central and north-central areas of the district (probabilities around 0.6–0.7). Low gradient desert dominates the western and northwestern portions of the district, and these areas are also generally closer to roads, which results in lower probability of javelina occurrence (around 0.4–0.5); Figure 1). Our ability to detect collared peccaries increased with the proportion of time cameras were operational and increased with deployment personnel experience. With experienced personnel, estimated detection probability, or the probability of detecting a javelina if present, was 0.40 (95% credible interval [CI] = 0.28, 0.68).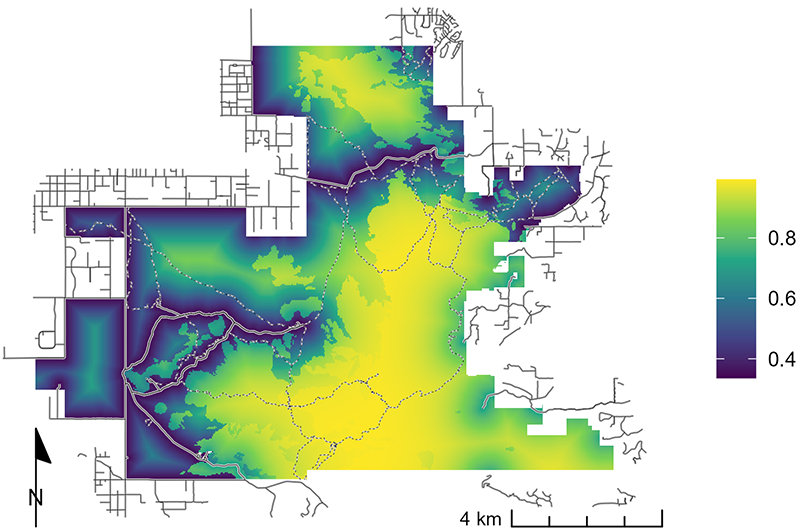
NPS
Desert cottontail
In 2023, none of our potential covariates explained where desert cottontails (Sylvilagus audubonii) were mostly likely to occur within the Tucson Mountain District. Overall, occurrence probability across the park for desert cottontails was 0.59 (95% credible interval [CI] = 0.08, 0.96). Overall detection probability for desert cottontails was 0.66 (95% CI = 0.11, 0.94), with detection probability increasing over the course of the sampling period and increasing with the proportion of time cameras were operational.
Mule deer
In 2023, mule deer (Odocoileus hemionus) were more likely to use lower elevation areas and areas farther from roads in the Tucson Mountain District of Saguaro National Park (Figure 2). Peaks and ridgelines in the southern and southeastern portion of the district had the lowest predictions of mule deer occurrence (probabilities around 0.2–0.3) with occurrence increasing in the lower elevations along the slopes. Mule deer were most likely to occur (probabilities around 0.6–0.8) in the low elevations in the west, central, and northern portions of the park, with the highest estimates in areas of low elevation that were also farther from roads (probability around 0.8; Figure 2). Our ability to detect mule deer decreased with day of the year (within the sampling period from 12 January to 22 February 2023) and increased with deployment personnel experience. Overall detection probability across the park for mule deer with experienced deployment personnel was 0.49 (95% credible interval [CI] = 0.40, 0.59).
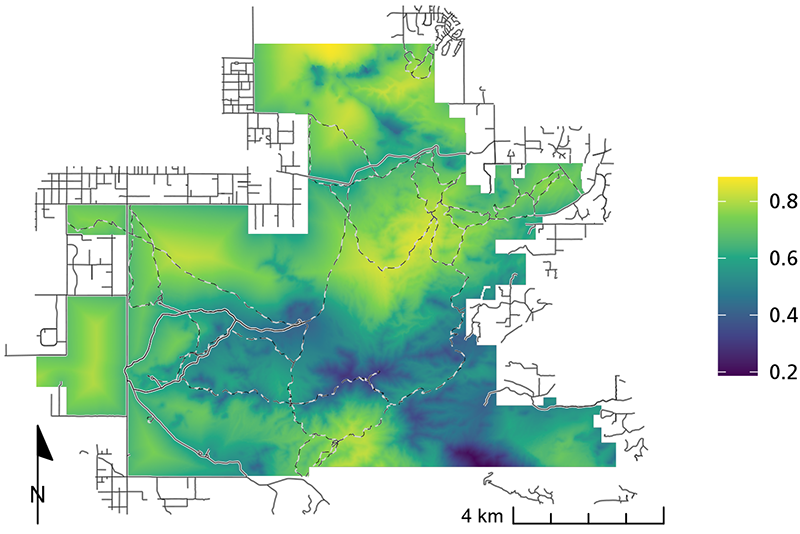
NPS
Black-tailed jackrabbit
Occurrence probabilities of black-tailed jackrabbits (Lepus californicus) were higher near park boundaries (boundaries that did not adjoin other protected land, such as Tucson Mountain Park to the south) and in areas that were relatively flat (i.e., low slope) and far from washes. These factors combined to predict the highest probabilities of black-tailed jackrabbit occurrence along the western areas of the Tucson Mountain District (Figure 3). The west and northwest portions of the district are flat and near the somewhat irregular park boundary, which led to occurrence probability estimates > 0.75. In contrast, much of the southeastern and central portions of the district contain hills and foothills with steeper gradients that are farther from the park boundaries. Jackrabbits are less likely to use these areas, and our predictions of occurrence probability were generally less than 0.5 (Figure 3).
Our ability to detect jackrabbits peaked near the midpoint (end of January and beginning of February) of the wildlife sampling period and, surprisingly, decreased with deployment personnel experience (Figure 4). Overall detection probability across the park for black-tailed jackrabbits with experienced deployment personnel was 0.45 (95% credible interval [CI] = 0.27, 0.63). It should be noted that the 247 photograph detections of unknown jackrabbits were not included in the analysis of black-tailed jackrabbits as they could not be identified to species.
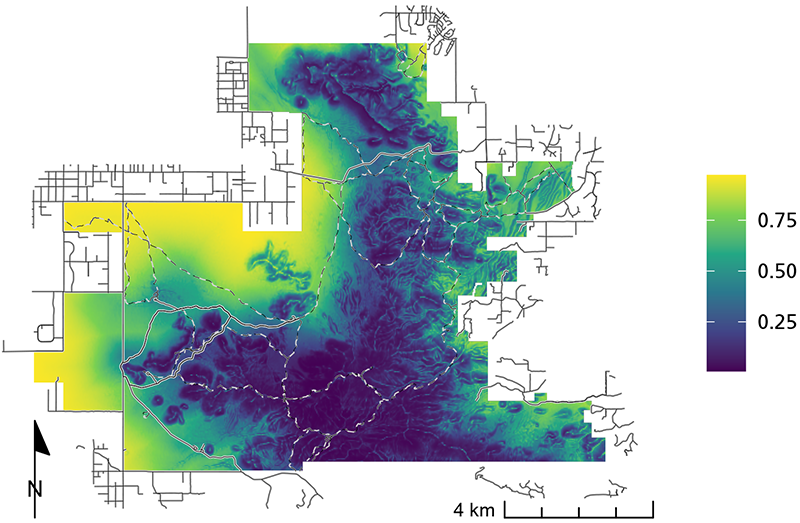
NPS
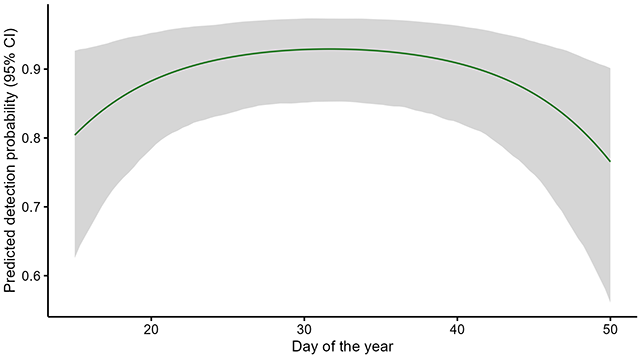
NPS
Coyote
In 2023, coyotes (Canis latrans) were more likely to occur in lower elevations of the Tucson Mountain District (Figure 5). Overall occurrence probability across the park for coyotes was 0.64 (95% credible interval [CI] = 0.41, 0.87), with < 25% of areas above 1,000 m (3,281 ft) likely used by coyotes in January and February. Our ability to detect coyotes was moderate (0.44 mean; 95% CI = 0.28, 0.68) and detection decreased as day of the year increased (within the 12 January to 22 February sampling period).
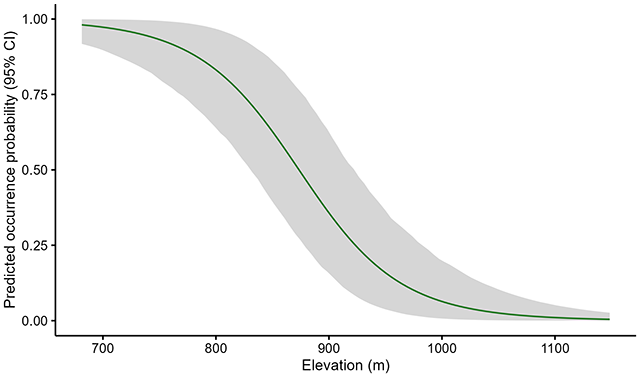
NPS
Gray fox
In 2023, mean occurrence probability of gray foxes (Urocyon cinereoargenteus) was 0.60 (95% credible interval [CI] = 0.45, 0.75). Gray foxes were more likely to occur in southerly and westerly facing areas and in areas far from trails. Combining these effects suggests that gray foxes were most likely to occur in the far western and southeastern portions of the Tucson Mountain District (Figure 6). The western, southeastern, and far northern portions of the district are mainly the farthest from trails and had high predicted probabilities of gray fox occurrence (> 0.7). The complex topography of the hills and foothills in the majority of the district, particularly in areas bisected by trails, resulted in areas with alternating high and low occurrence probabilities (Figure 6). Detection probability of gray foxes across the park averaged 0.49 (95% CI = 0.38, 0.61) and varied over time, with detection probability peaking around the middle of the sampling period.
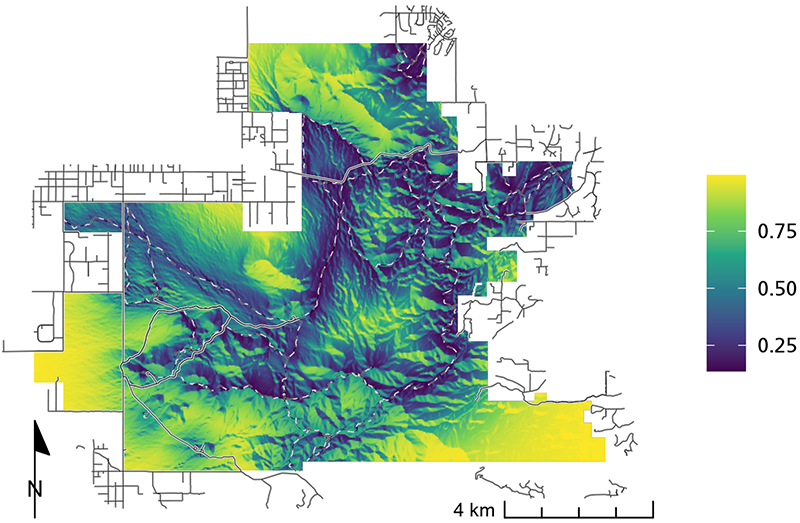
NPS
Bobcat
Although there were few detections of bobcats (Lynx rufus) in 2023, we were able to fit an occupancy model, albeit a complicated one. Aspect and a combined factor of vegetation and landform predicted that bobcat occurrence was highest on south-facing slopes in the northern and southwestern areas of the Tucson Mountain District (Figure 7). Predictions of bobcat occurrence were highest (> 0.5) on south-facing slopes, particularly in the northern, central, and southwestern portions of the district. The vegetated areas of the low desert in the southwestern portion of the district also had fairly high occurrence predictions (probabilities around 0.4–0.5). The hilly and often jojoba-dominated areas in the southeastern portion of the district had fairly low predictions of bobcat occurrence (Figure 7). Detection probability of bobcats increased over time and with deployment personnel experience. Overall bobcat detection probability across the park with experienced deployment personnel was 0.33 (95% credible interval [CI] =0.17, 0.50).
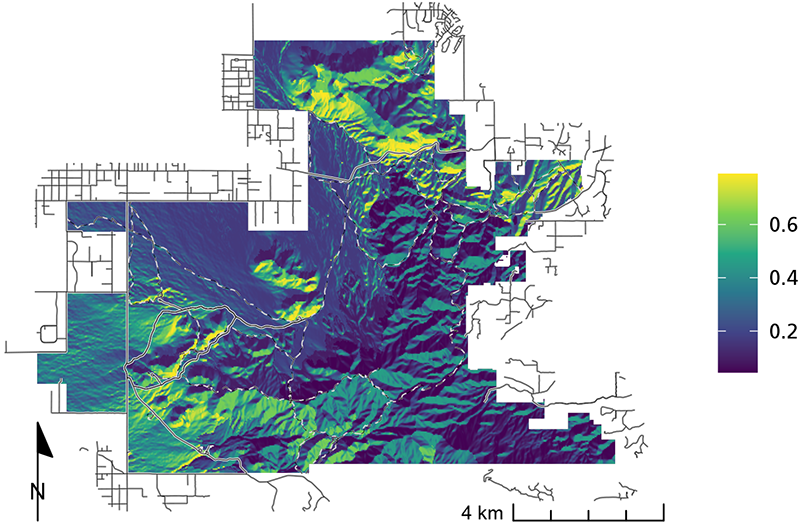
NPS
Literature Cited
Beery, S., D. Morris, and S. Yang. 2020. Efficient pipeline for camera trap image review [computer software]. Available at http://github.com/agentmorris/MegaDetector.
Brashares, J. S. 2010. Filtering wildlife. Science 329: 402.
Carroll, C. 2010. Role of climatic niche models in focal-species-based conservation planning: assessing potential effects of climate change on northern spotted owl in the Pacific Northwest, USA. Biological Conservation 143: 1432–1437.
Chen, C., and others. 2022. Global camera trap synthesis highlights the importance of protected areas in maintaining mammal diversity. Conservation Letters 15(2).
Doser, J. W., A. O. Finley, M. Kéry, and E. F. Zipkin. 2022. spOccupancy: an R package for single-species, multi-species, and integrated spatial occupancy models. Methods in Ecology and Evolution 13: 1670–1678.
Hubbard, J. A., E. C. Dillingham, and A. Buckisch. In prep. Terrestrial mammal monitoring protocol implementation plan for the Sonoran Desert Network. National Park Service, Sonoran Desert Network.
Ivan, J. S., and E. S. Newkirk. 2016. CPW Photo Warehouse: a custom database to facilitate archiving, identifying, summarizing and managing photo data collected from camera traps. Methods in Ecology and Evolution 7: 499–504.
MacKenzie, D. I., J. D. Nichols, G. B. Lachman, S. Droege, J. Andrew Royle, and C. A. Langtimm. 2002. Estimating site occupancy rates when detection probabilities are less than one. Ecology 83: 2248–2255.
Post, E., J. Brodie, M. Hebblewhite, A. D. Anders, J. A. K. Maier, and C. C. Wilmers. 2009. Global population dynamics and hot spots of response to climate change. Bioscience 59: 489–497.
Please cite this report as
McIntyre, C., J. McGeverly, and E. Zylstra. 2024. Wildlife Monitoring in Saguaro National Park, Tucson Mountain District: 2023. Sonoran Desert Network, National Park Service, Tucson, Arizona.